
The integration of Artificial Intelligence (AI) into Battery Energy Storage Systems (BESS) has become a game-changer for the energy sector. With the increasing reliance on renewable energy sources and the urgent need for more efficient energy storage, AI-powered energy storage systems are emerging as a key innovation. Through machine learning and data analytics, BESS efficiency can be maximized, providing a more reliable and cost-effective solution for both utilities and businesses.
What is AI-Powered Energy Storage?
Battery Energy Storage Systems (BESS) have been a cornerstone in enabling renewable energy integration, allowing for the storage of solar or wind energy for later use. However, as energy consumption patterns become more dynamic, traditional methods of managing BESS are proving to be less effective. This is where AI and machine learning come into play.
How AI Enhances BESS Efficiency: AI technologies, particularly machine learning algorithms, are applied to BESS systems to predict energy usage patterns, optimize battery performance, and enable predictive maintenance. This leads to:
- Longer Battery Lifespan: AI helps in optimizing charging and discharging cycles, preventing overuse or degradation of batteries.
- Improved Energy Distribution: AI systems can intelligently manage energy output, reducing energy waste and enhancing the system’s overall efficiency.
- Predictive Maintenance: Machine learning algorithms predict faults before they occur, reducing downtime and maintenance costs.
Chart 1: Comparison of BESS Performance with and Without AI Integration
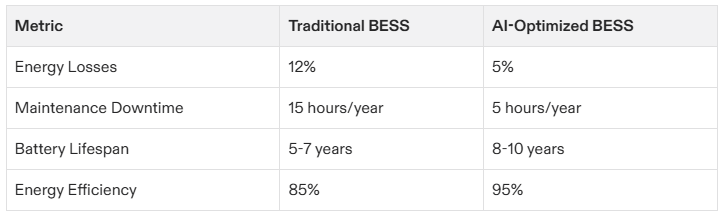
The Role of Machine Learning in Optimizing BESS
Machine learning plays a crucial role in optimizing the operations of energy storage systems. By analyzing massive volumes of data in real-time, machine learning models can make decisions that significantly improve the efficiency and lifespan of BESS.
Key Applications of Machine Learning in BESS:
- Load Prediction and Optimization: Machine learning algorithms predict energy usage patterns, helping to store energy at optimal times. This ensures that energy is available when needed and reduces the risk of energy shortages.
- Battery Health Monitoring: By analyzing data from sensors, machine learning can detect early signs of battery wear or failure. This allows operators to schedule maintenance or replace batteries before a complete failure occurs.
- Dynamic Energy Management: AI algorithms can adjust the storage and release of energy dynamically, ensuring that the BESS is always functioning at peak efficiency. This is especially important for variable energy sources like solar or wind, which produce fluctuating amounts of energy.
Chart 2: Impact of Machine Learning on Battery Life and Efficiency
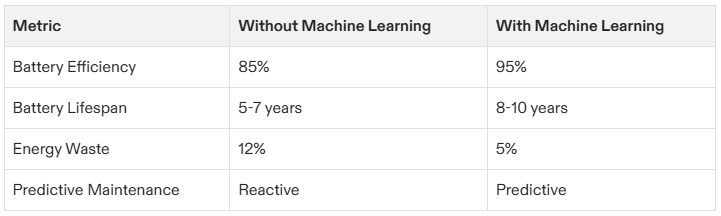
Real-World Applications of AI in Energy Storage
Across the globe, businesses and utilities are already adopting AI-powered BESS solutions to optimize energy storage and lower operational costs. These applications are paving the way for smarter, more efficient energy systems.
Case Study 1: Utility-Scale BESS Optimization
A large utility company in California has integrated machine learning algorithms into its BESS, improving energy storage and grid stability. The AI system predicts peak energy demand times, ensuring that energy is stored when demand is low and released when demand peaks, reducing grid strain and improving cost savings.
Case Study 2: Commercial Energy Storage
A retail chain in the United Kingdom utilizes AI-based energy storage to manage its on-site solar power generation. The system intelligently stores excess solar energy during the day and uses it to power the store at night, reducing energy costs by 20%.
How AI Improves Energy Storage with Predictive Analytics
The power of AI lies not only in its ability to optimize storage in real-time but also in its predictive capabilities. By analyzing historical data and identifying patterns, AI systems can forecast energy demand and battery performance far in advance, allowing for proactive management.
Benefits of Predictive Analytics in BESS:
- Energy Demand Forecasting: AI models can predict fluctuations in energy demand, allowing for the preemptive release or storage of energy.
- Preventive Maintenance: Predicting when components will require maintenance allows for scheduling repairs before breakdowns occur.
- Optimized Charging Cycles: AI can adjust charging cycles to ensure batteries are charged during periods of low electricity demand or when renewable energy generation is at its peak, reducing operational costs.
Challenges and Opportunities for AI in Energy Storage
While AI-powered energy storage presents incredible opportunities, there are challenges to consider. These systems require high-quality data, substantial investment in infrastructure, and ongoing monitoring to ensure accuracy and efficiency.
Challenges:
- Data Quality and Availability: AI models require high-quality data from BESS systems, and without it, predictions and optimizations may not be accurate.
- High Initial Investment: Implementing AI-powered energy storage systems requires an initial investment, especially for infrastructure and AI integration.
Opportunities:
- Smart Grid Integration: AI-powered BESS can be integrated into smart grids, allowing for seamless energy distribution and improving grid stability.
- Cost Reduction Over Time: While the initial investment is high, AI-driven optimizations lead to lower energy costs and longer-lasting systems, making the ROI highly favorable in the long run.
Chart 5: AI Adoption vs. Traditional Energy Storage Adoption
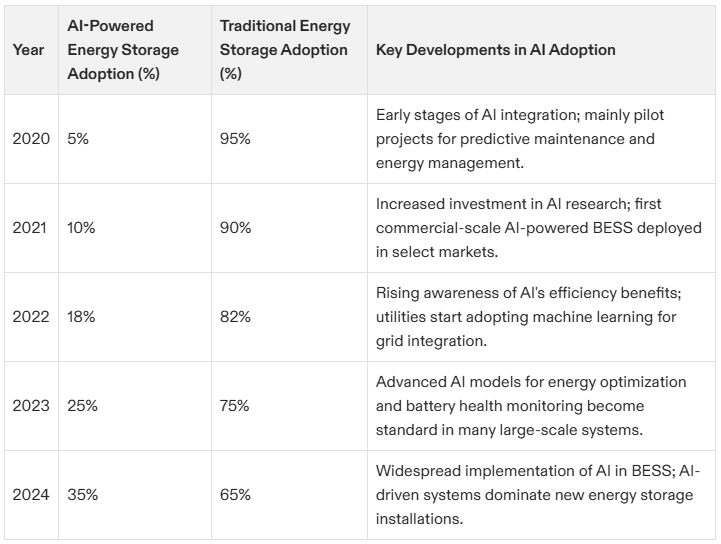
FAQs
1. How does AI improve the efficiency of energy storage systems?
AI-powered algorithms analyze energy usage patterns and storage conditions, optimizing charge and discharge cycles to enhance the overall efficiency of energy storage systems (BESS). This leads to better energy management and reduced operational costs.
2. What are the benefits of machine learning for Battery Energy Storage Systems (BESS)?
Machine learning enables BESS to predict energy demand, anticipate failures, and optimize energy usage, which improves system lifespan and performance. It helps in reducing energy waste and increasing reliability.
3. Can AI optimize the charging and discharging cycles in a BESS?
Yes, AI optimizes both the charging and discharging processes by learning from real-time data, adjusting battery operation to maximize energy retention while minimizing degradation, ultimately enhancing battery lifespan.
4. How does machine learning help in predictive maintenance for energy storage systems?
Machine learning models use historical and real-time data to predict potential system failures before they happen. This enables proactive maintenance, reducing downtime and extending the life of BESS systems.
5. Can AI integration help with the integration of renewable energy into storage systems?
AI plays a key role in managing renewable energy integration into BESS by forecasting energy generation patterns, adjusting storage capacities, and ensuring optimal distribution of renewable energy across the grid, thereby maximizing efficiency.
Conclusion: The Future of AI in Energy Storage
AI and machine learning are driving the next wave of innovation in energy storage. With the ability to predict energy demand, optimize storage, and reduce maintenance costs, AI-powered BESS systems are quickly becoming the standard for both utilities and businesses. The potential for cost savings, operational efficiency, and improved sustainability is immense, and as these technologies continue to evolve, their impact on the energy sector will only increase.
If you’re looking to maximize the efficiency of your energy storage system, consider implementing AI-powered solutions. Contact Sunpal Energy to learn how we can help integrate machine learning into your BESS, enhancing performance and reducing costs.